AI LLM’s: The X-Factor Innovation Unlocking the SMB Market for the Financial Services Sector
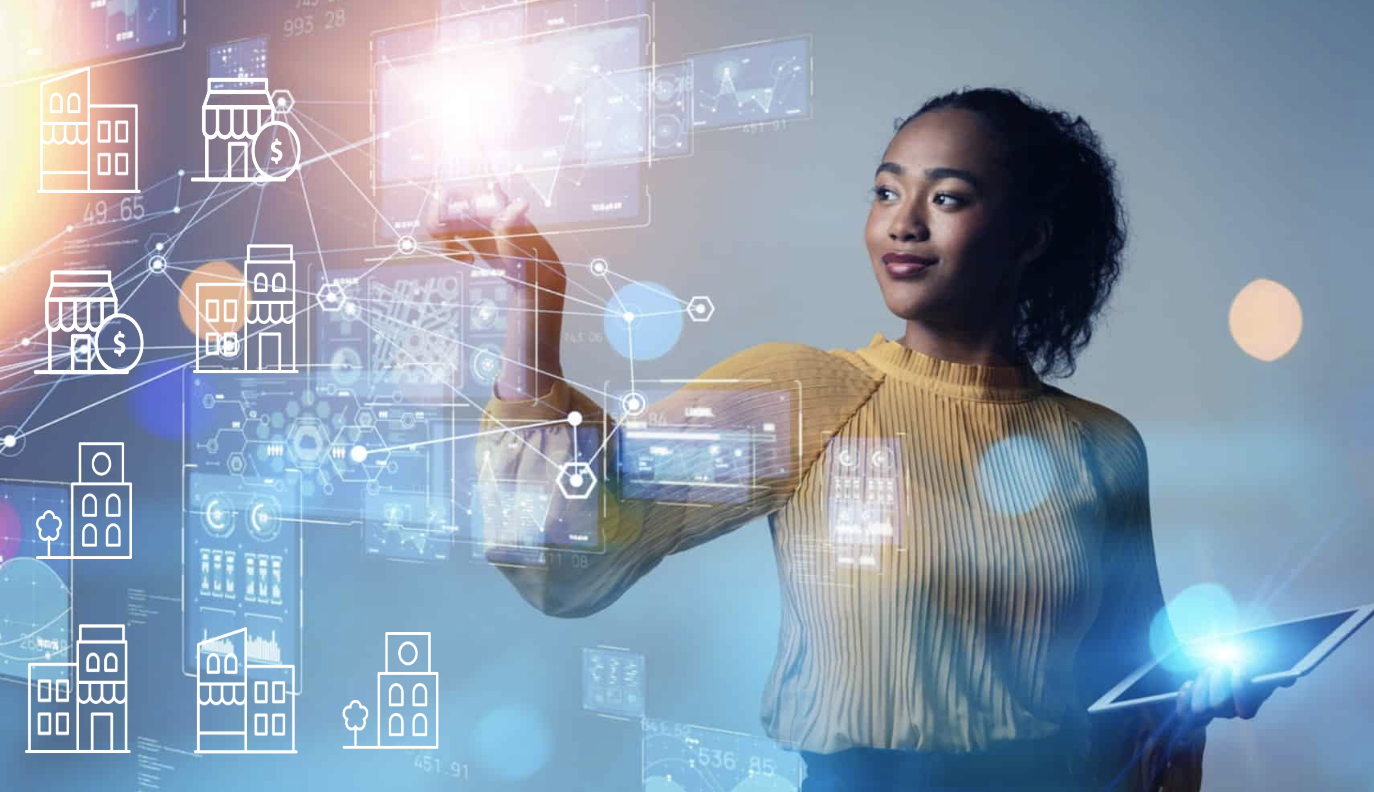
Executive Summary
The use of AI as a business tool has captured the attention and imagination of organizations around the world curious about deploying it to deliver meaningful business value. Key innovations in AI, large language models (LLMs) and machine learning (ML) have now made it possible to unlock key insights into one of the most challenging sectors: privately held small and medium sized businesses (SMBs).
These technology breakthroughs will completely overhaul the way innovation leaders and sales & marketing teams at banking, financial and insurance organizations identify, understand and track great-fit SMBs and convert those leads into customers.
Using AI that applies techniques at the frontier of machine learning to monitor the public web, companies can identify, track and measure critical events, signals, activities and interactions any SMB has online through its website, social media accounts, news articles, videos, and public sentiment. This allows financial institutions and insurers to forge a comprehensive look into how that company is doing, what it’s in need of, and what it might look for in terms of future products and services.
Instead of appearing as just another face in the crowd to the key stakeholders of small businesses and startups, insurance and financial companies can target and segment SMBs uniquely with a considerable amount of information about the company and its most valuable people, giving it a unique competitive advantage.
This sort of AI-enabled deep data analysis can lead to multiple benefits, including an increase in sales & marketing efficiency, reduction of costs, faster lead-to-customer conversion, increased customer lifetime value (LTV), lower risk profiles, more process automation efficiency, and more.
Introduction
Big businesses might dominate the headlines and the stock market in the US, but small businesses are the lifeblood of the nation’s economy. There are more than 33 million of them in the US - accounting for 99.9% of all businesses, they are responsible for creating 63% of all new jobs over the past three decades, and despite the ravages of COVID-19, there were more than 5.4 million new business applications filed in the US in 2021. Small businesses are everywhere, from single-person proprietorships to 250-person thriving companies that have found their niche and are experiencing staggering growth as a result.
All those companies compose a staggering amount of diversity along an infinite number of industries, but they all have a few things in common. Namely that they require financial institutions to set up their business accounts, get loans, and acquire business credit cards, and they all need at least the bare minimum of business insurance, and some multiple kinds of it, to be compliant with their geography and industry, as well as protected from extraordinary events.
For the sales and marketing teams of insurance firms and financial institutions, the challenge of efficiently identifying the fastest-growing SMBs and engaging them can be daunting. Public companies are required to publish business data and information in annual and quarterly reports. This public data provides an easily accessible source of truth for segmentation, targeting and general sales & marketing purposes. SMBs represent a very different challenge. Critical data about their infrastructure, financial health, key decision makers, location data and growth trajectories is scattered and unstructured. Collecting, sorting, normalizing and interpreting this data so sales & marketing teams can make sense of it to acquire new and grow existing SMB customers has either proved to be inefficiently manual or simply ignored.
Every problem needs a solution, especially this one. Recent advances in the powerful new technologies of Artificial Intelligence (AI) and Large Language Models (LLM) are proving to efficient and effective solutions.
The combined availability of Artificial Intelligence (AI), Machine Learning (ML) and Large Language Models (LLMs) puts marketing and sales teams at the brink of a new era in the historically challenging SMB market where they can actively and reliably collect:
- Higher-quality SMB data
- A deep understanding of the activities of the SMBs
- Verified SMB data that can be used to confidently model & segment
Combined, these three dynamic points will deliver a quintet of key benefits, notably:
- Increased bookings & lead-to-customer conversion
- Lower risk profiles and an overall lower risk of default
- Reduction of costs
- Increase in sales and marketing efficiency
Problem Statement
Marketers, innovation leaders and industry experts alike face significant challenges in targeting and engaging SMBs. Their size is always a factor, but analyzing their “corporate breadcrumbs” and gleaning insights from that digital exhaust are also tripping points.
A common hangup comes from the inability to be resource-effective due to the size of the SMB. Researching small businesses can be a pain-staking task that can feel like a wild goose chase. Being able to sort out what a maximum spend might be from the SMB could make it cost-prohibitive to even start a file on them.
Once that hurdle is overcome, there’s still the serious issue of engaging each SMB with the right type of content. Not just the type, but the timing, the language, and the channel as well. A big factor in this search is that SMBs are often atypical of what we consider a company to look like, in terms of ownership, the way they communicate with their customers and potential customers, and what stage of the business cycle they are in. Traditional media is usually not the way to engage, meaning there has to be a very nuanced approach which will likely change from one SMB to the next. Having wide swathes of data for some companies and a scant crop for others also makes it more difficult to have a consistent, sustainable approach to rapidly explore and engage with multiple SMBs.
Timing is everything, so if your organization can’t intersect the needs of an SMB and comes across with the wrong pitch, the wrong tone, or the wrong channel, the likelihood of getting a second half is not very good.
Unlike bigger companies that are frequently releasing data to the public, the media, their various trade associations, their stockholders, and more, smaller businesses aren’t actively involved in the release of public information, except when they have good news to announce, and even then, they might not have it go too far beyond their own websites and a few social media pages. In normal circumstances, that might mean having someone from your marketing/sales team attempting to track a business every single day of the year on every single possible outlet they use, trying to find the perfect moment to send an email, start a conversation, dial a number, or even walk into the business to say hello.
The data is hard to quantify on your own, and there aren’t large receptacles of public knowledge out there waiting to be devoured by crawlers and analytic software. There are simply too many small businesses doing too many different things to be able to pull data traditionally from them all and somehow collate it into actionable movements forward. Sure, you might go door to door through a strip mall or a business park and gather information the old fashioned way, but what good does that do once you’ve spent an hour or two exhausting those leads and you’re ready to move on to the next group of small businesses? What happens when you have the bandwidth for legions of customers, but you have no proven way of identifying and quantifying the needs of millions of businesses that are out there?
It’s as much a scaling problem as it is a workflow problem, and requires a leap forward in combining AI with data analysis in order to net real results that can be the difference between a sale and a strikeout for your company.
The Power of AI and Large Language Models
Let’s start with some introductions so that we’re all on the same page. We know what the sales and marketing teams of our banks and insurance companies need, but how does AI provide them with that solution?
Large Language Models (LLMs) are a class of AI that employ neural networks, simplified versions of the ones that wire our brains, that can make intelligent analysis of text to understand their content and track different relationships, such as words in a sentence or a social media post. Given enough data points, LLMs can predict and generate content of their own as well.
LLMs need three things to work:
- Language understanding with semantic analysis
- Resource crawling
- Maintenance of a deep topic ontology
Semantic Analysis: Semantic AI allows machines to more successfully understand the intent of terms. It’s the reason Google shows you a picture of Elvis Presley when you search “rock music” instead of a boulder with a treble clef on it. Semantic AI is used universally in classifying content, in search result autocompletion, by chatbots and voice assistants like Alexa and Siri, and to develop personalized results and recommendations on sites like Amazon and so forth.
The web crawlers classify the context of the text it is looking at, making decisions on what sort of organization the business is, what its products and services are, who its key people are, what topics are most important to the company, what jobs it has advertised, and what growth signals are being expressed. The language models are trained offline in controlled environments with smaller volumes of data so that they can understand the language that actually matters in a story, a social media post, or in the copywriting on a company website. Doing it offline allows the models to be tested, trained, and retested until they become strong enough to be deployed online with a high rate of success.
Resource Crawling: This is pretty much what it sounds like, in that the web crawlers start on a site reading content and will follow the links that are associated with that page to gain more insight into what the text is referring to, picking out the valuable information and leaving the rest without having to crawl through it. This saves enormous amounts of time and energy that would otherwise be dedicated to the computing power needed to make such an endeavor happen.
Topic Ontology: Ontology means a formal description of knowledge that exists as a set of concepts within a domain and the relationships that exist between them. That doesn’t necessarily clear up how they work in LLM. In layman's terms, topic ontology means that the AI is developing concepts about the things it is crawling, enabling it to define relationships and make connections that pair items together and begin forming analysis and suggestions as a result. You can imagine the topic ontology like you would imagine the scaffolding that holds up a building. You can’t see it from the outside, but without it, the building would collapse immediately. These concepts can include everything from business units to position roles to products and services inside of an organization.
Using the power of AI and LLMs can capture immense amounts of data about any company, including SMBs, without having to attempt to have their personnel fill out a survey or try to get it via cold call. The model allows a company to really get an insider look at how a company is doing, and how it is being seen by its customer base and the general public. Perhaps even more importantly, it can reveal a company’s timeline of its business lifecycle and allow interested third parties to bear witness to things like product launches, expansions, times of expanded hiring, revenue growth, mergers and acquisitions (M&A), and other events and trends that signify what stage the business is in and give financial and insurance marketing and sales representatives key insight into how they could specifically help the company meet its future goals.
For instance, say your LLM returns key information on a startup business that has hit it big with sales of a certain niche product that has seen it blast off. The company’s own social media presence is blowing up, full of complimentary reviews, retweets, likes, and questions about when the product will be available in other geographic locations. The LLM will pick up all of this information, along with the fact that company is continuously posting new job positions on its job board and also chew up the transcript of a news story in which the CEO of the company is quoted as saying that they are already exploring scaling-up plans to reach as many markets as possible.
This is the perfect collection of data needed to help a financial institution make the decision to contact the company and offer to help them scale up to as many new locations as possible with fluidity and flexibility to make it happen without breaking the bank. Or an opportunity for an insurance company to give the SMB a presentation on how to adapt to different laws in different jurisdictions about insurance and how the company can make the transition a smooth one. It’s a bit like omnichannel marketing, although somewhat in reverse. AI modeling is allowing third party companies to take the journey along with the small business they want to target, seeing what is working for them, where they are succeeding, and where they need help along the way.
Key Business Lifecycle Events and Signal Monitoring
As discussed, SMBs represent a large market segment with significant opportunity for banks, financial institutions and insurers. However, their small size, private ownership and the unstructured nature of their data make them historically difficult to efficiently acquire and service from a sales & marketing perspective.
AI technology has the power to change this by identifying and monitoring key signals about SMBs at unprecedented scale.
A “signal” captures a fundamental change in a business such as advertising new jobs, confirmation of a company’s business model & structure (re: E-commerce) and more. That signal should be demonstrable, evidence-based and can be captured via the public web. Monitoring signals allows service providers in finance and insurance to grasp what lifecycle stage a business is in and what challenges they might be seeking solutions to. Let’s take a look at some of these signals that can be identified and monitored with AI.
New Product or Service Launch: When an SMB makes any sort of move - launching a new product or service, making the news, or unveiling a press release or social media post, your service needs to know about it.
Job Advertisements: When jobs come available at an SMB, it means either there is expansion in the company or there is turnover that might mean turmoil that needs financial or insurance solutions.
New Offices: Nothing says big changes are afoot for an SMB more than expanding to new offices, new locations, or a move to a bigger facility. Tracking news, posts, and articles on the subject gives your company a leg up.
Hiring News: These can often fall under the radar unless you’re tracking social media and LinkedIn channels and accounts. New hires can mean expansion or reorganization.
E-Commerce: E-Commerce is a huge component of most SMBs, many of whom do not have a traditional brick-and-mortar location and rely completely on online sales to drive their business models. This takes the form of shopping carts, payments, and ecommerce platforms.
Growth, Awards & Positive News: Every company likes to show off, and this is a perfect way to track everything that is going positive for the SMB from news and articles to posts and social media notice. A good way to track sentiment and measure non-traditional growth.
Imports, Exports, US & International Trade: Not every company is limited to doing business domestically. This lens allows you to see what a certain company is shipping in, shipping out, and in which countries they do business.
Headcount Trend: Track social media posts, articles, blogs, and press releases to see if the company is getting larger or smaller over time.
Product & Services Taxonomy: Each product and services a company offers can be cataloged and tracked to reveal insights about the SMB’s business model. These insights go much further than traditional NIACS, SIC or standard industry classifications to deliver a nuanced, modern understanding of what makes a company tick.
Funding: Has the SMB set up a funding protocol page in an attempt to raise money for an expansion, a scale-up or some other program.
Supply Chain & Partnership: A business is only as strong as its partners, clients, suppliers, and other companies that serve it along the value chain. Tracking & understanding these dynamics can reveal key growth opportunities.
Layoffs & Negative News: Monitoring negative news sentiment and layoffs can have significant impact on the viability of a small business and if it makes sense to invest resources in doing business with them.
M&A: Is the company moving forward via merger? Some firms move so quickly they gobble up the competition; others are such a threat that they devoured first. Which camp is the firm you are tracking in?
Active Company: Some businesses have an amazing website but actually aren’t actively operating. Make sure a firm is up and running before investing resources.
Leveraging AI to identify and monitor these key signals has already proven to make a significant impact in the financial sector.
Case Study: AI Signal Monitoring for SMBs in Action
In a recent collaboration with the Business Card Analyst & Innovation Team at a Fortune 500 bank, AI monitoring of key SMB insights was put to the test. The bank’s team was interested in finding a solution to address four specific value drivers for the business:
- Predict when a new customer SMB might need a new credit line or when an existing customer needs an increase in credit (measured as “Booking Rate”)
- Improve risk models to identify new & existing customers with a lower chance of delinquency or default
- Improve the efficiency of sales & marketing efforts by focusing financial and human capital resources on the “right” SMB accounts.
LeadGenius and Glass.ai turned loose their AI research capability to deep read the websites, news feeds, public sentiment, social channels and other public web resources on a priority segment of SMBs identified by the bank. Fourteen different signals were identified and monitored with the following four key signals revealing the most significant lift:
- New Office Openings (“Evidence New Office”)
- 12 Month Company Headcount Growth Trend (“Increase in Headcount”)
- Current Total Number of Jobs Advertised (“Evidence Jobs Advertised”)
- Hiring News Events & Articles (“Hiring Signals”)
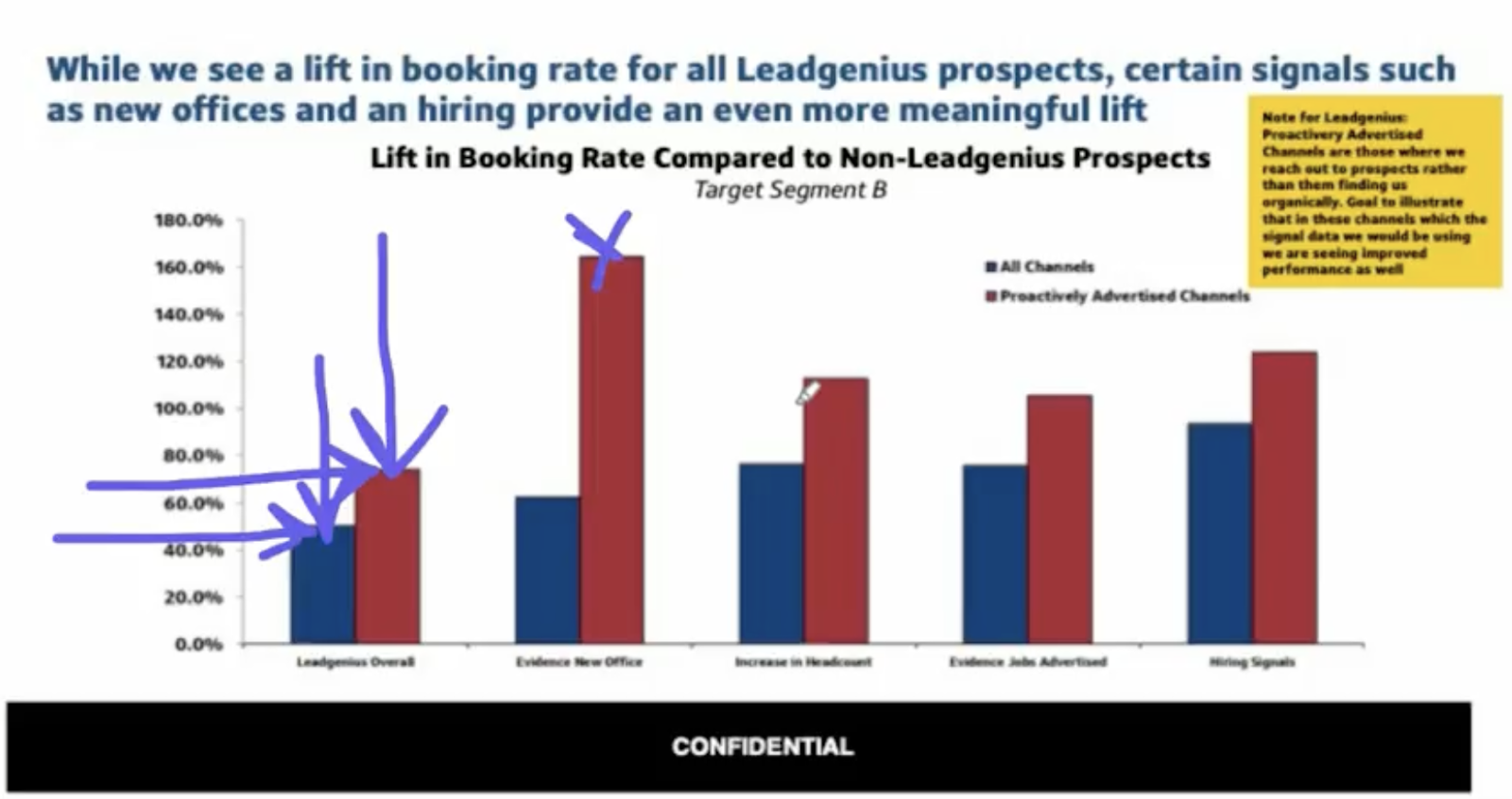
EXHIBIT A.
Exhibit A shows significant business impact in helping acquire new customers:
- Overall, companies with signals present had a 50% lift in booking rate across all acquisition channels and an almost 80% lift in booking rate on proactive advertising channels (direct sales & marketing, digital & display advertising)
- “Evidence New Office” was the most impactful signal, contributing to a 160% increase in booking rate on proactively advertised channels
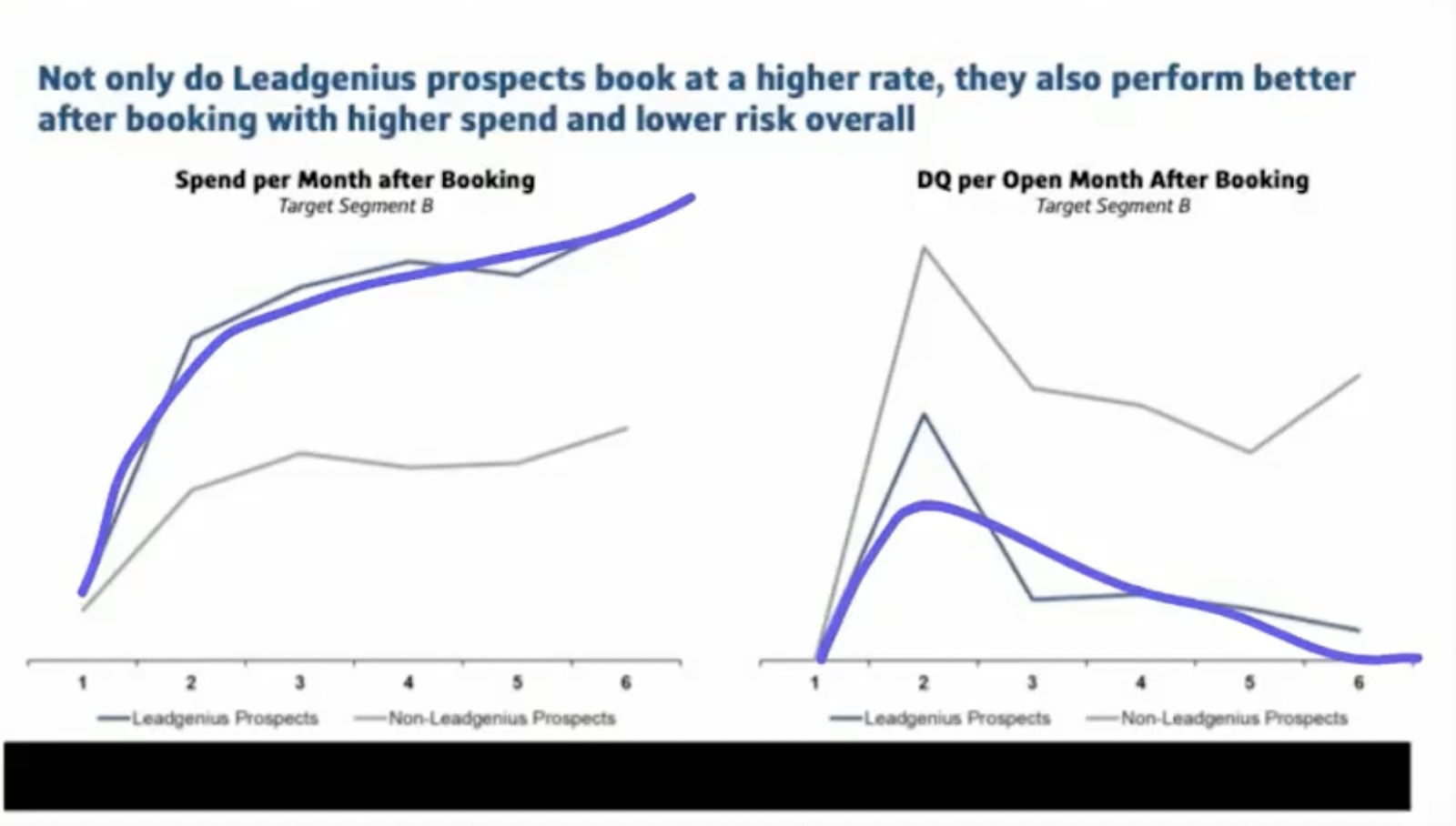
EXHIBIT B.
Exhibit B shows significant business impact with existing customers:
- SMBs with signals present were significantly more likely to spend more over time after becoming a customer than those without
- Companies with signals present had significantly fewer delinquencies over a 6 month period than those without
“Not only did these insights contribute to higher booking rates, they also revealed accounts with higher spend and lower overall risk,” said the company’s commercial data business director.
Advances in AI are proving to make an unprecedented impact on how financial institutions, banks and insurance companies go to market acquiring, servicing and growing SMB customers.
Other Benefits for Financial Services and Insurance Firms
Adapting any new technology requires an adjustment period and some growing pains, regardless of how proficient it is. However, the benefits inherent for financial services and insurance firms are numerous and can start taking hold almost immediately. They include:
Reduce cost & increase efficiency: Sales and marketing orgs should not be data analysts or researchers. Their time is best spent winning new customers and growing existing ones. They should have the best data available at their fingertips at the moment they’re ready to use it. Using AI to identify and track SMBs has the power to make this possible. Teams can know which SMBs to target and why, then make educated decisions on how best engage a lead based on the likelihood of converting them into a customer.
Faster decision making & competitive advantage: In the 20th century, banks and financial institutions were waiting for months to get data analysis from the prior quarter or year, hoping to gain insight into what should happen next to stay in the black. AI and LLM reduce that lag time down to almost nothing given their collection and analysis powers. With markets saturated by heavy competition, being able to act faster than the “other guy” is a huge step forward to winning more business.
Faster compliance processes: Is that new client going to be worthy of a loan, a business credit card, or a comprehensive insurance policy? The answer to those questions used to involve significant paperwork and man-hours. By building an AI-powered case file on each SMB, orgs can see from an early point in the lifecycle whether or not a potential client is going to make the grade, and plan accordingly.
Conclusion
AI and LLMs are uniquely specific technologies that have the power to bridge the gap between the frustrations of traditional marketing and sales for financial institutions and insurance companies seeking to find new customers among small businesses. By harvesting massive amounts of data from the Internet and using intuitive algorithms to build comprehensive, analytical reports on small businesses, these models can provide marketing and sales staff with an incredible wealth of insight into companies that are looking for insurance and/or financial services on a very niche level based on their current spot in the business lifecycle. Having this information at hand is a huge linchpin in helping service providers identify points of potential collaboration in startup businesses around the world. These insights eliminate the need for cold calling and guessing and replace those antiquated techniques with an insider view of what makes a business and its leadership tick.
ABOUT LEADGENIUS & GLASS.AI
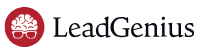
LeadGenius helps mid-market and enterprise revenue leaders acquire their best fit customers globally. LeadGenius uniquely sources account, contact and signal data on-demand and just-in-time using a proprietary blend of technology automation and human intelligence. LeadGenius is backed by some of the most prominent investors in the Silicon Valley including Sierra Ventures, Lumia Capital, Javelin Venture Partners and SJF Ventures.

Glass.ai is an AI research capability that deep reads the web to discover, understand and track the activities of millions of companies. Glass.ai technology reads web content from multiple sources across countries and languages. It is used by B2B corporates, governments, consulting and market research firms, universities and non-profits. These organizations use glass.ai to research sectors, find new clients, segment markets, and monitor millions of companies on growth signals and other indicators.